About
For centuries, the method of discovery—the fundamental practice of science that scientists use to explain the natural world systematically and logically—has remained largely the same. Artificial intelligence (AI) and machine learning (ML) hold tremendous promise in having an impact on the way scientific discovery is performed today at the fundamental level. However, to realize this promise, we need to identify priorities and outstanding open questions for the cutting edge of AI going forward. We are particularly interested in the following topics:
-
Solving grand challenges in structural biology
-
Scaling dynamical system modeling to millions of particles
-
Visualizing the unimaginable black hole
-
Incorporating physical insights to AI methods
-
Accelerating drug discovery pipeline
Follow Us
Please follow us on Twitter and LinkedIn for the latest news, or join us on the Slack for active discussions.
Invited Talks (In alphabetical order)
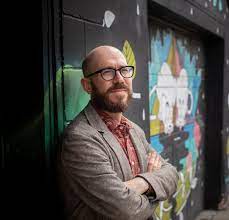
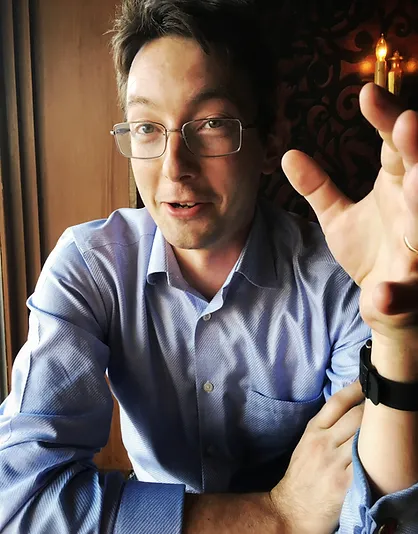
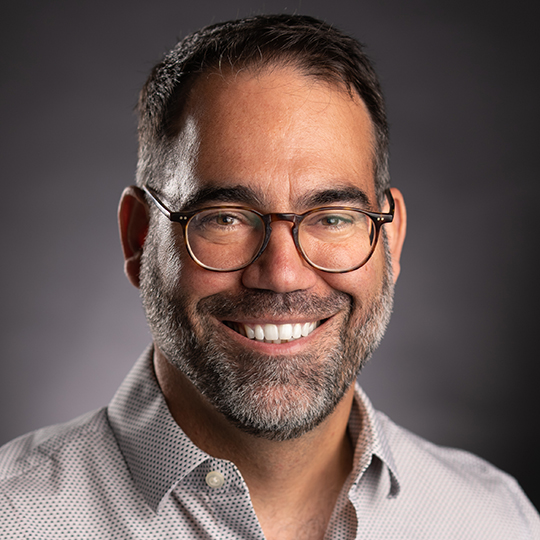
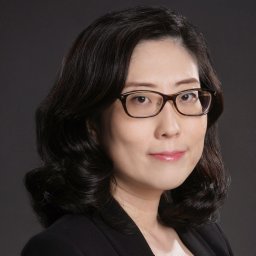
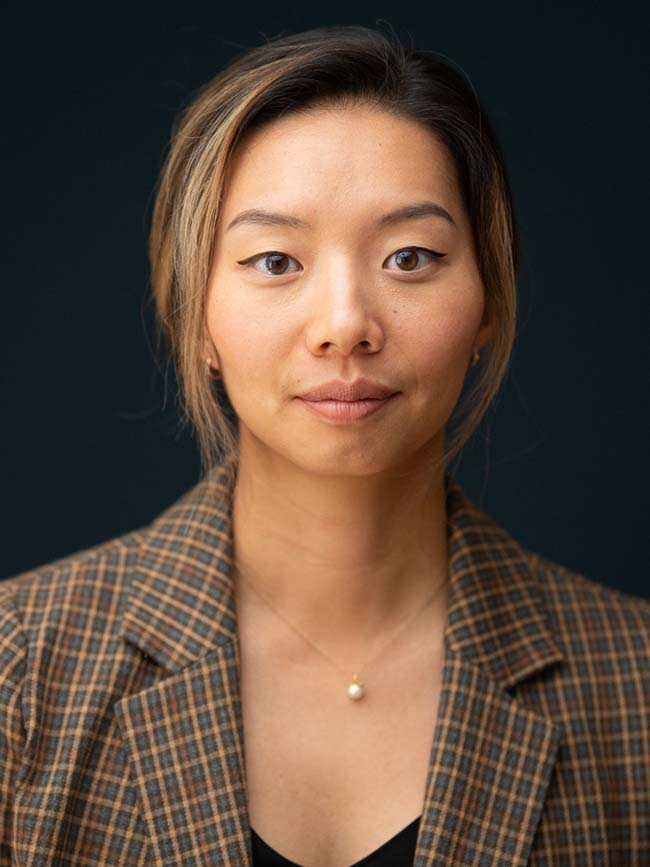
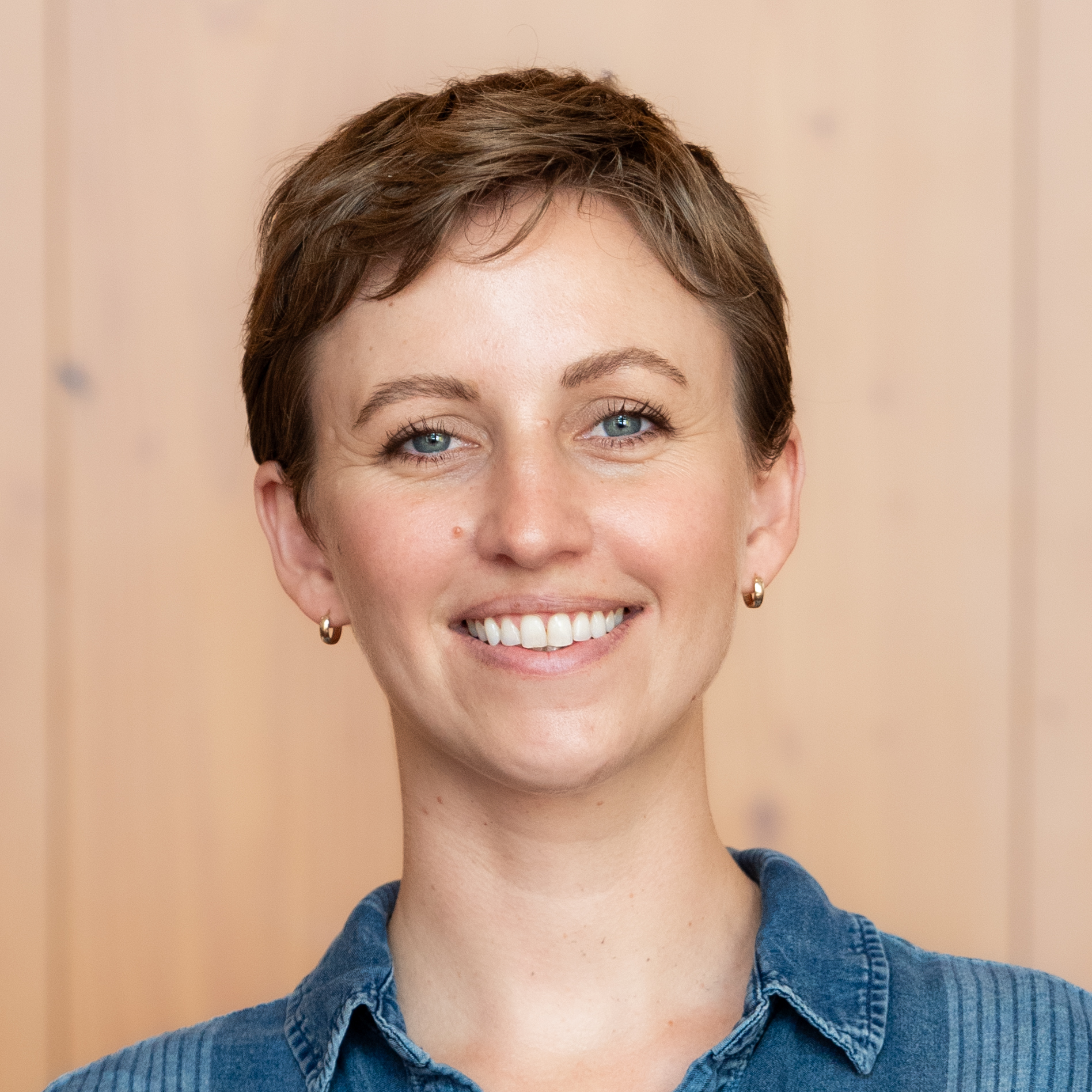
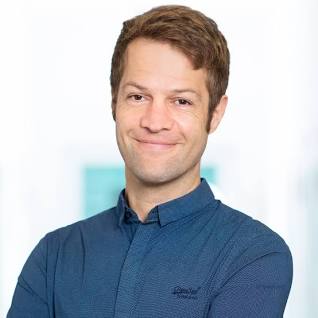
Panel: Using AI to Accelerate Scientific Discovery
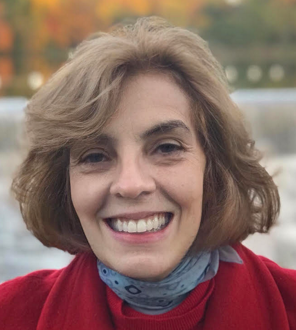
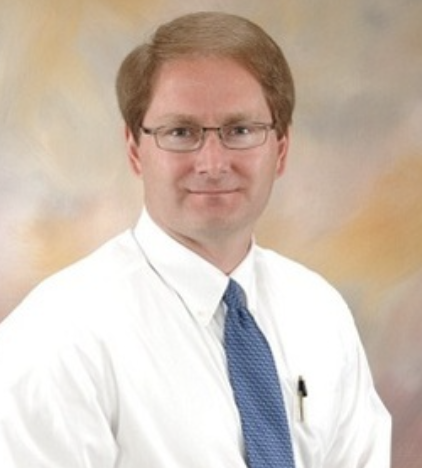
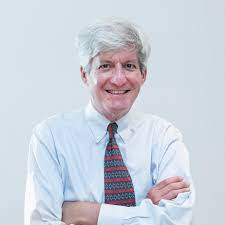
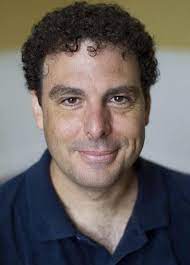
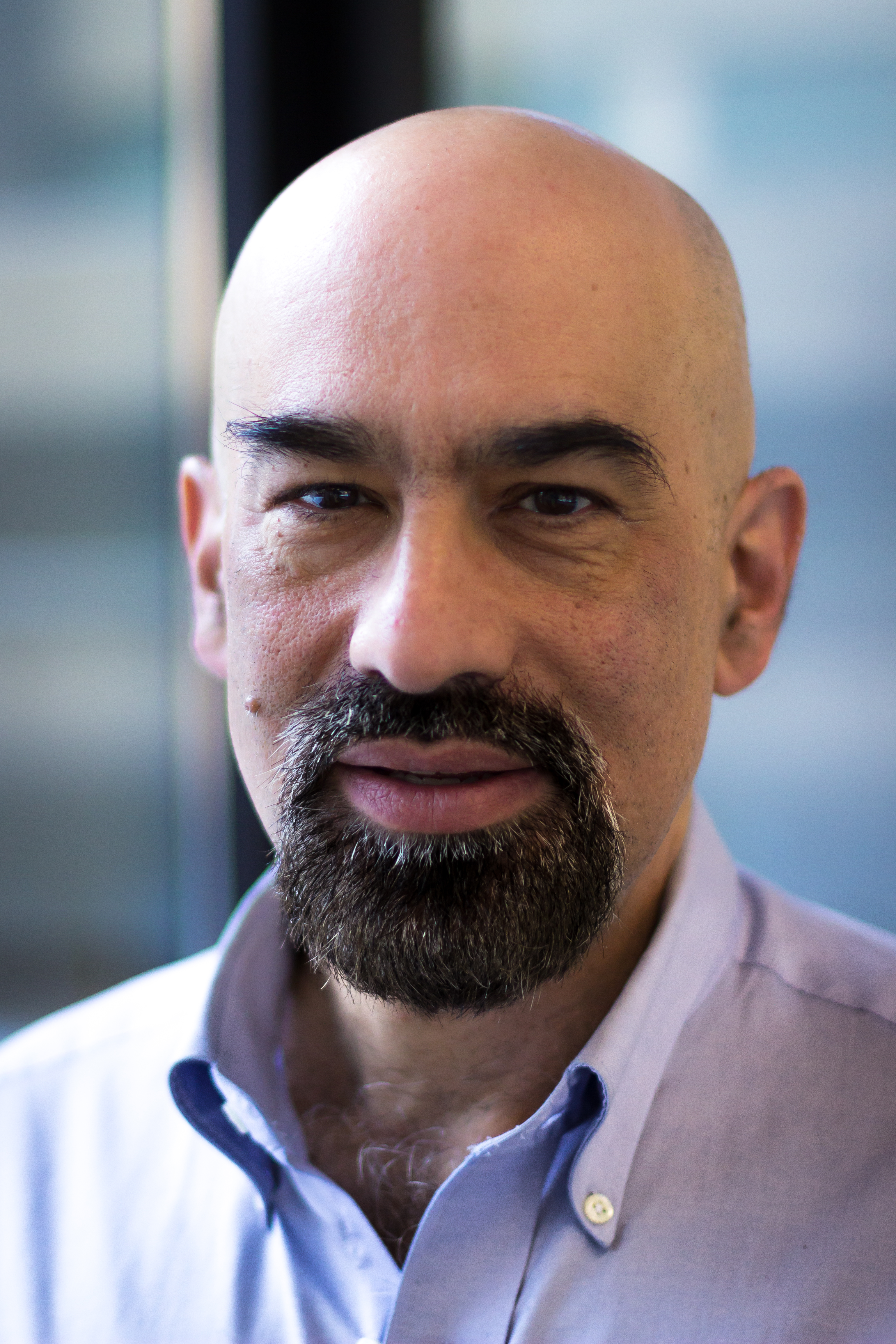
Open Catalyst Challenge
The Open Catalyst Challenge 2023 invites participants to help address the pressing challenges faced by the world due to energy scarcity and climate change. In this area, a critical problem is the discovery of new catalysts for driving efficient and carbon-neutral means for energy storage and conversion.
Over the past two years, the Open Catalyst Challenge has focused on the central task of relaxed (local minimum) energy prediction. This year’s task of determining the adsorption energy (global minimum) will require relaxed energy prediction as a subtask.
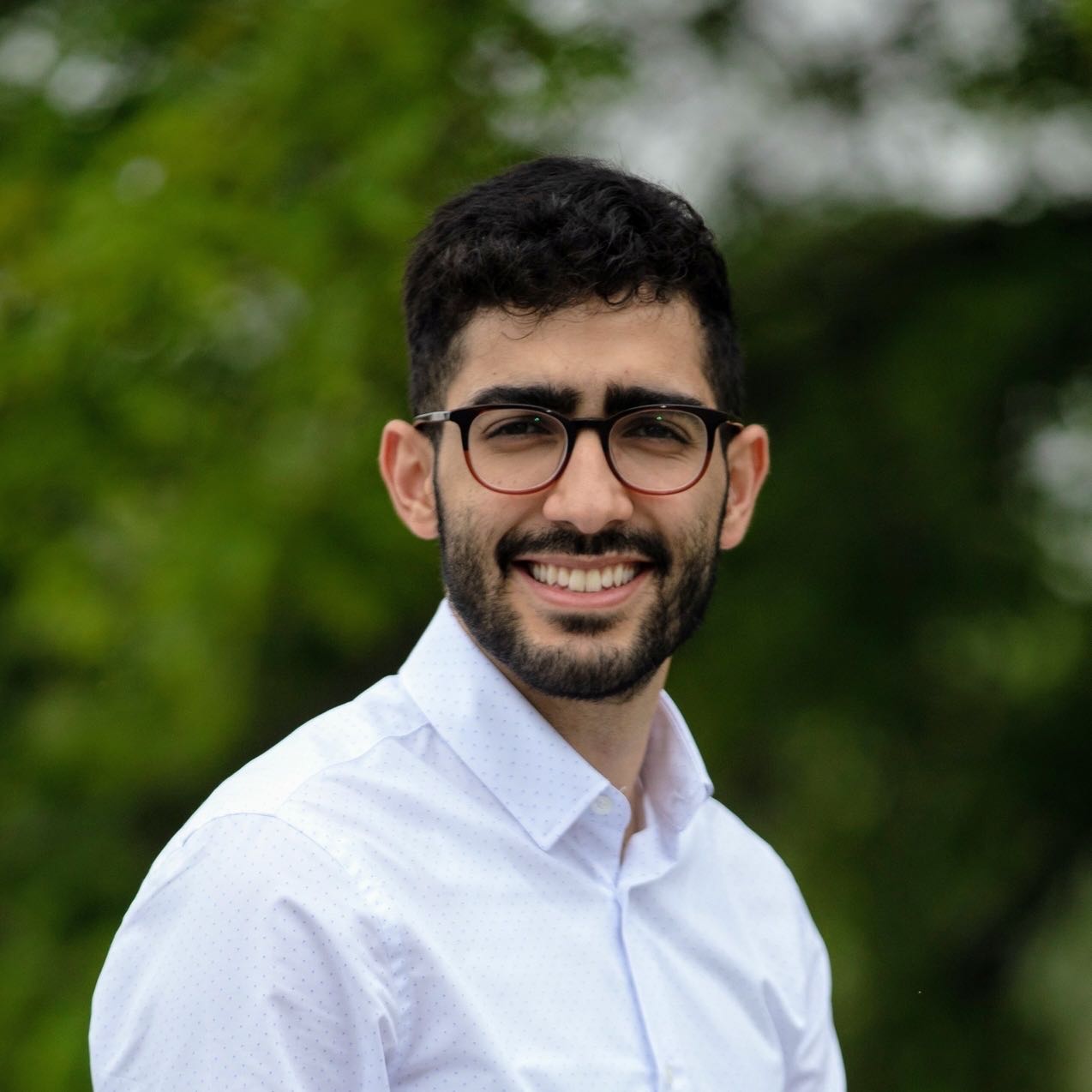
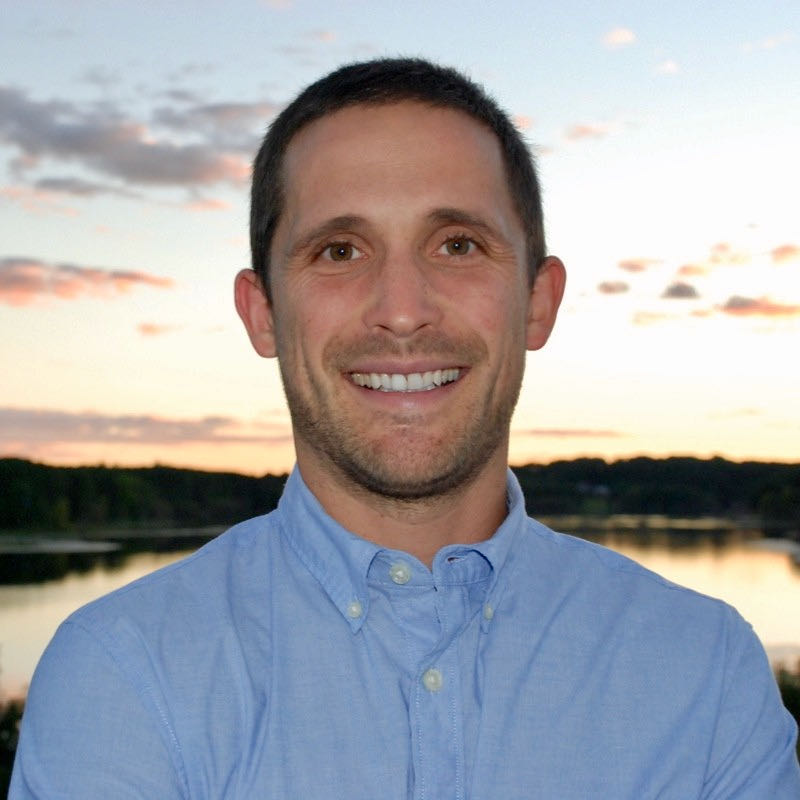
Important Dates (Anywhere on Earth)
- Abstract Submission Deadline: Sept 30, 2023
- Paper Submission Deadline: Oct 2, 2023
- Review Bidding Period: Oct 2-4, 2023
- Reviewer Deadline: Oct 24, 2023
- Acceptance Notification Date: Oct 27, 2023
- Workshop Date: Dec 16, 2023
Submissions
Please submit your paper in Openreview. Our workshop is nonarchival, the accepted papers will be posted on our website.
Organizers and Contact
Organizers are in alphabetical order. For any question, please contact ai4sciencecommunity@gmail.com.
Organizers
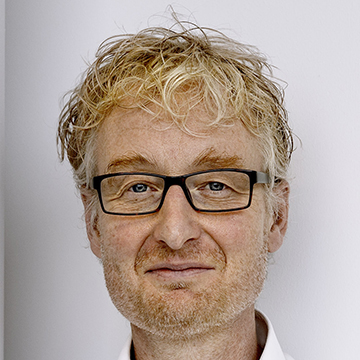

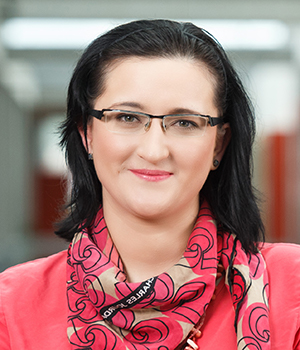
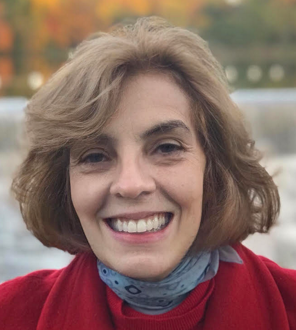
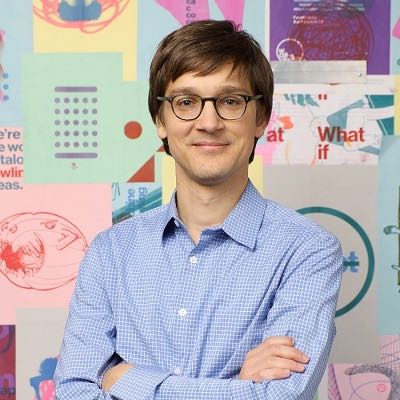
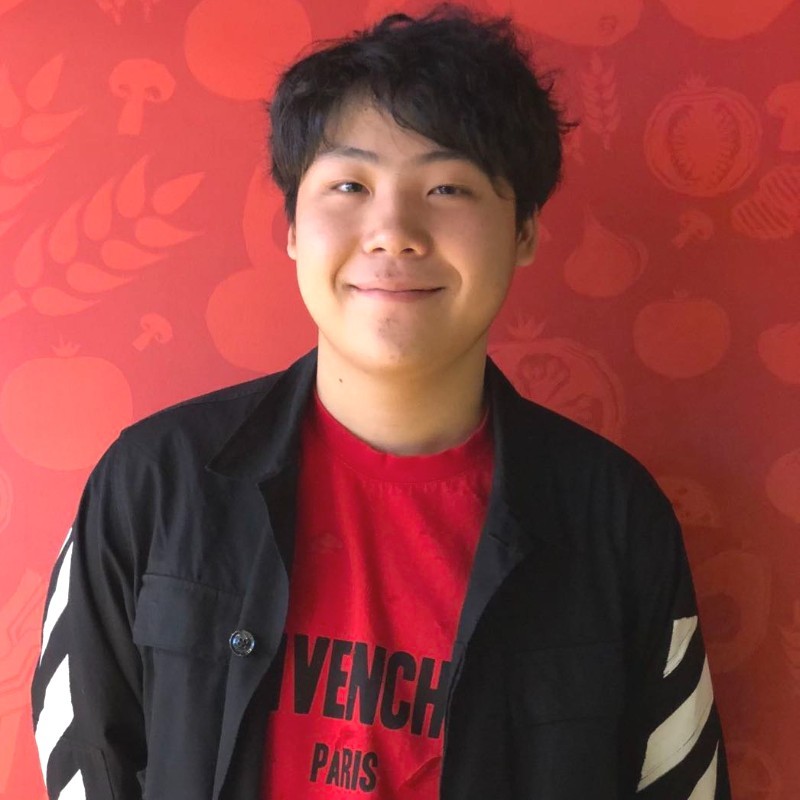
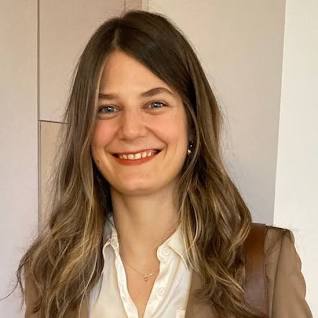
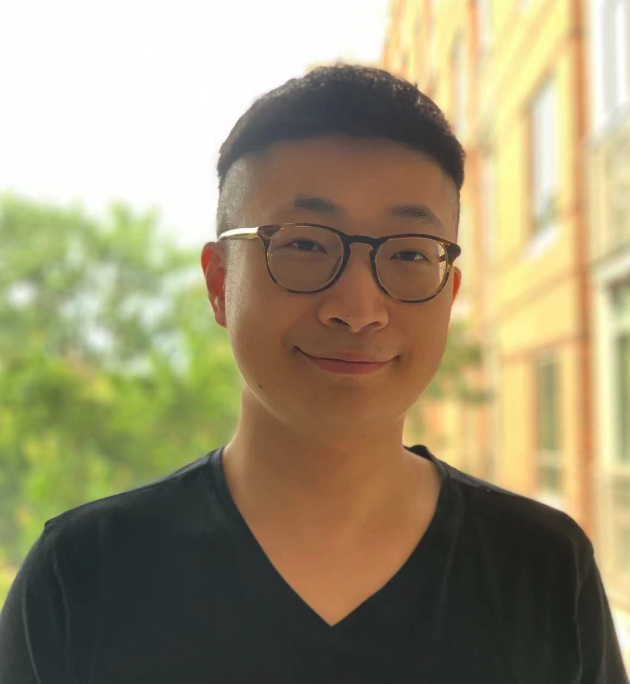
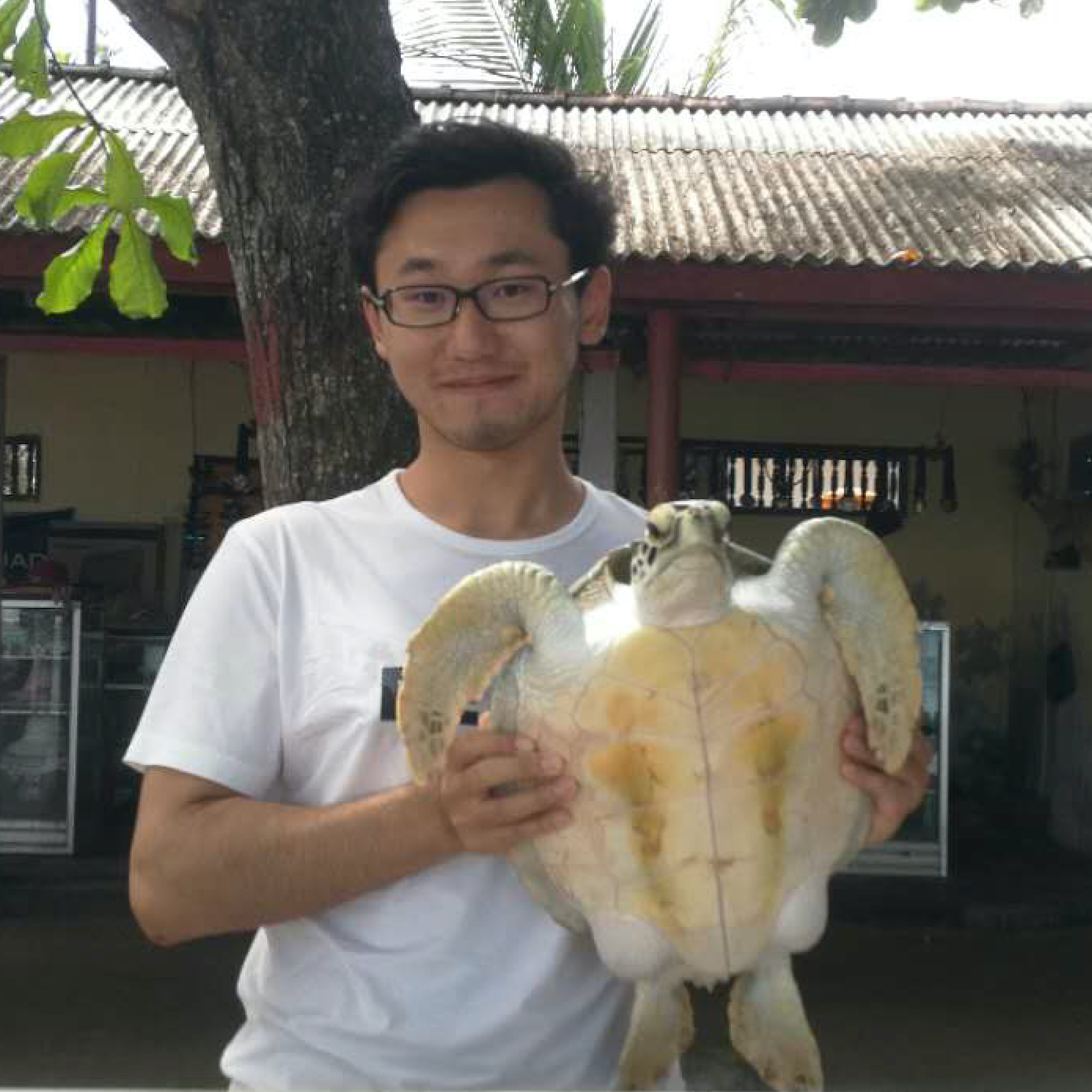
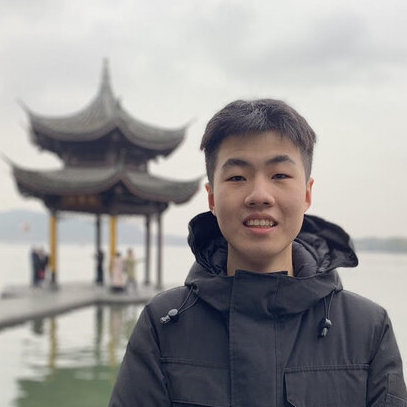
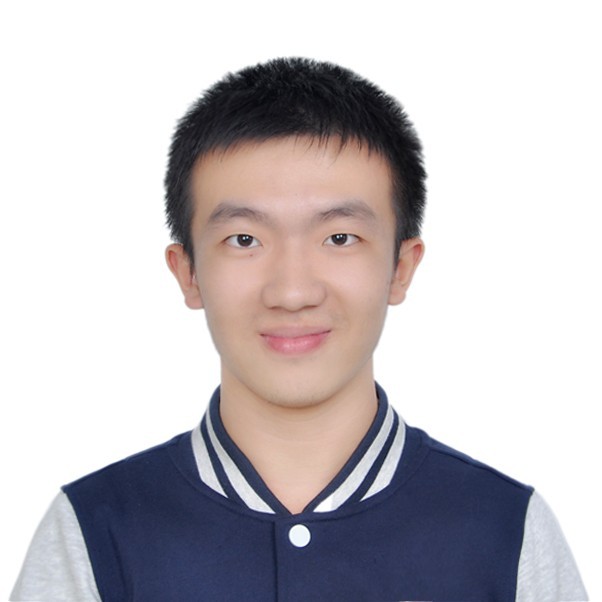
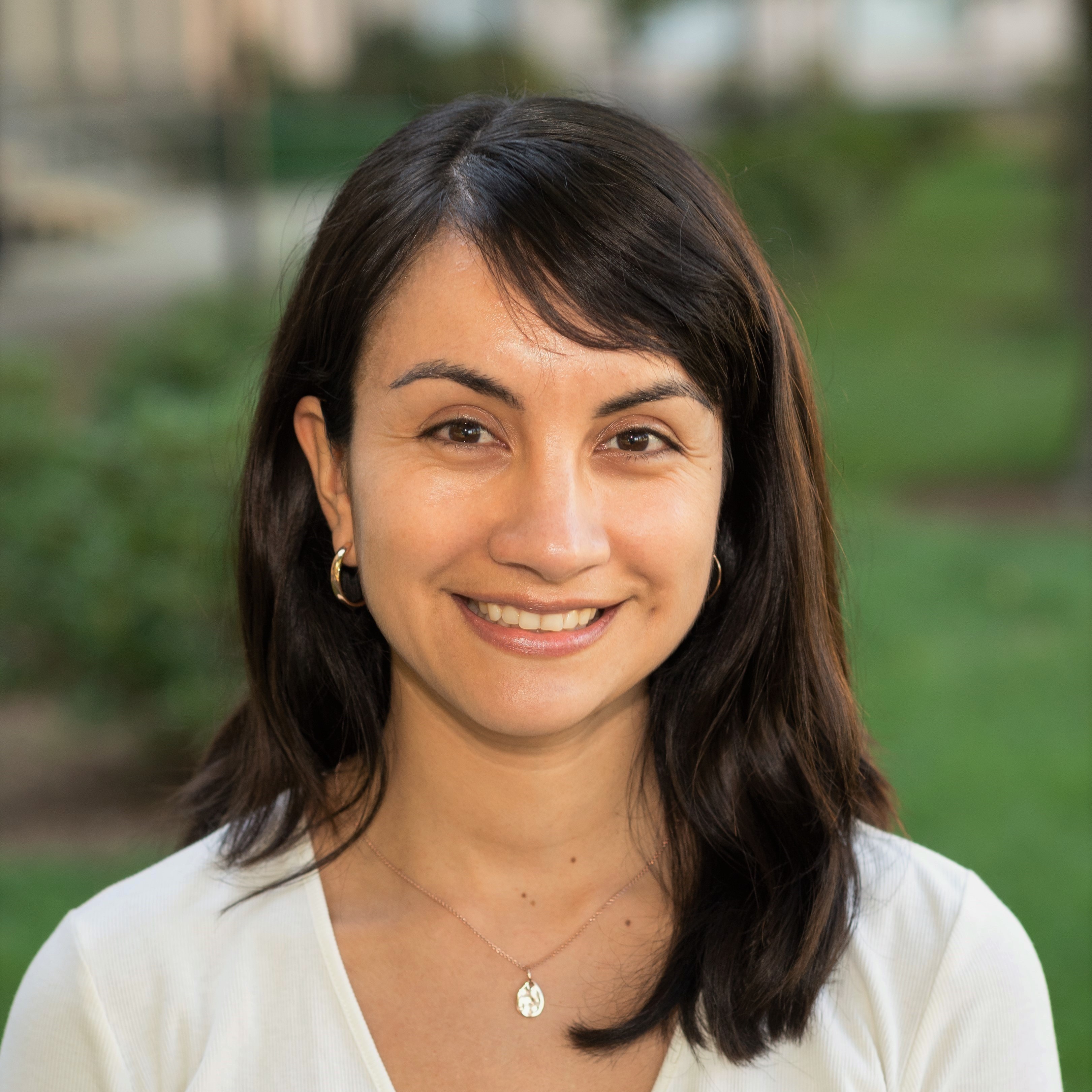
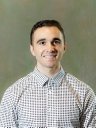
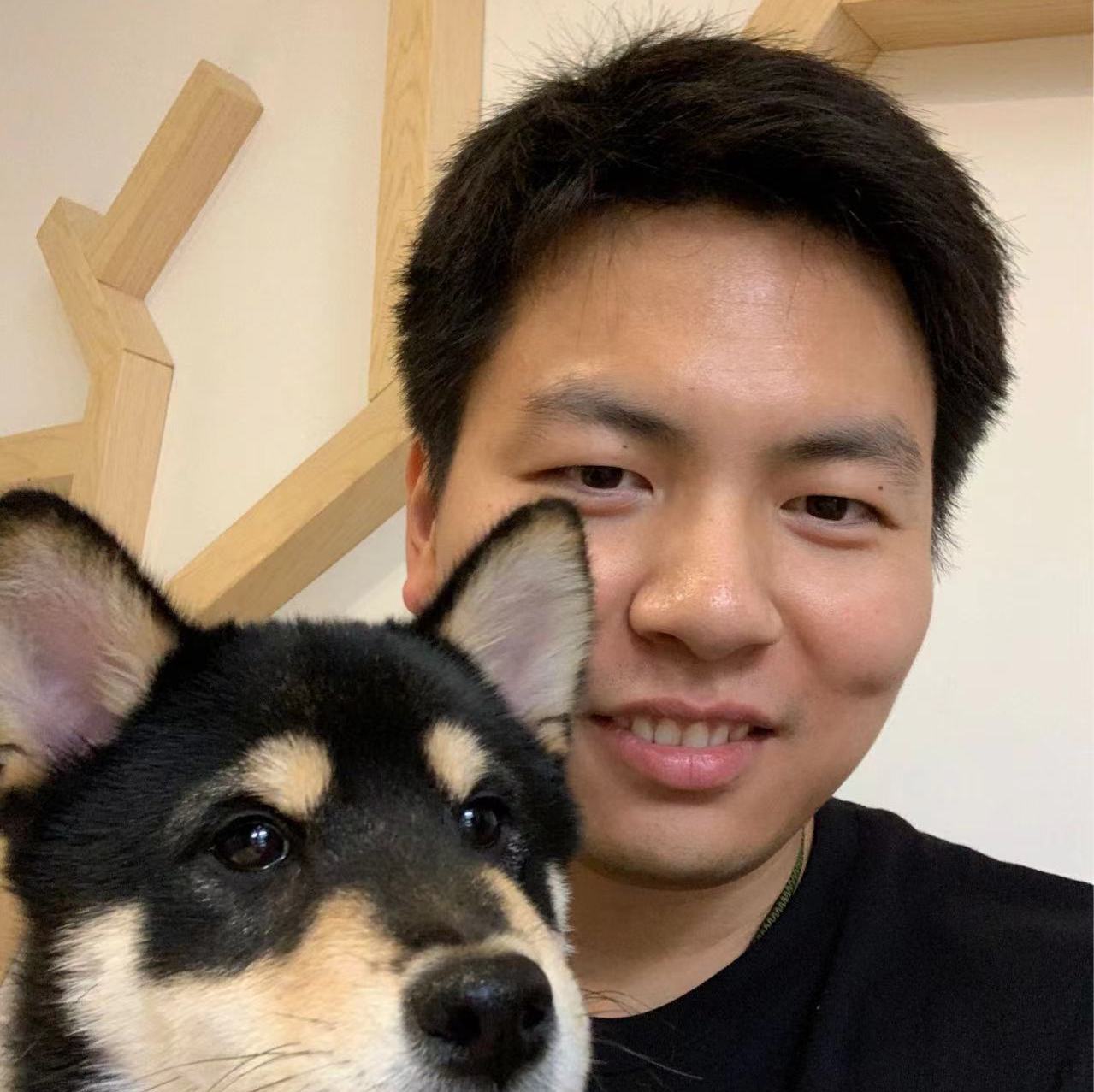
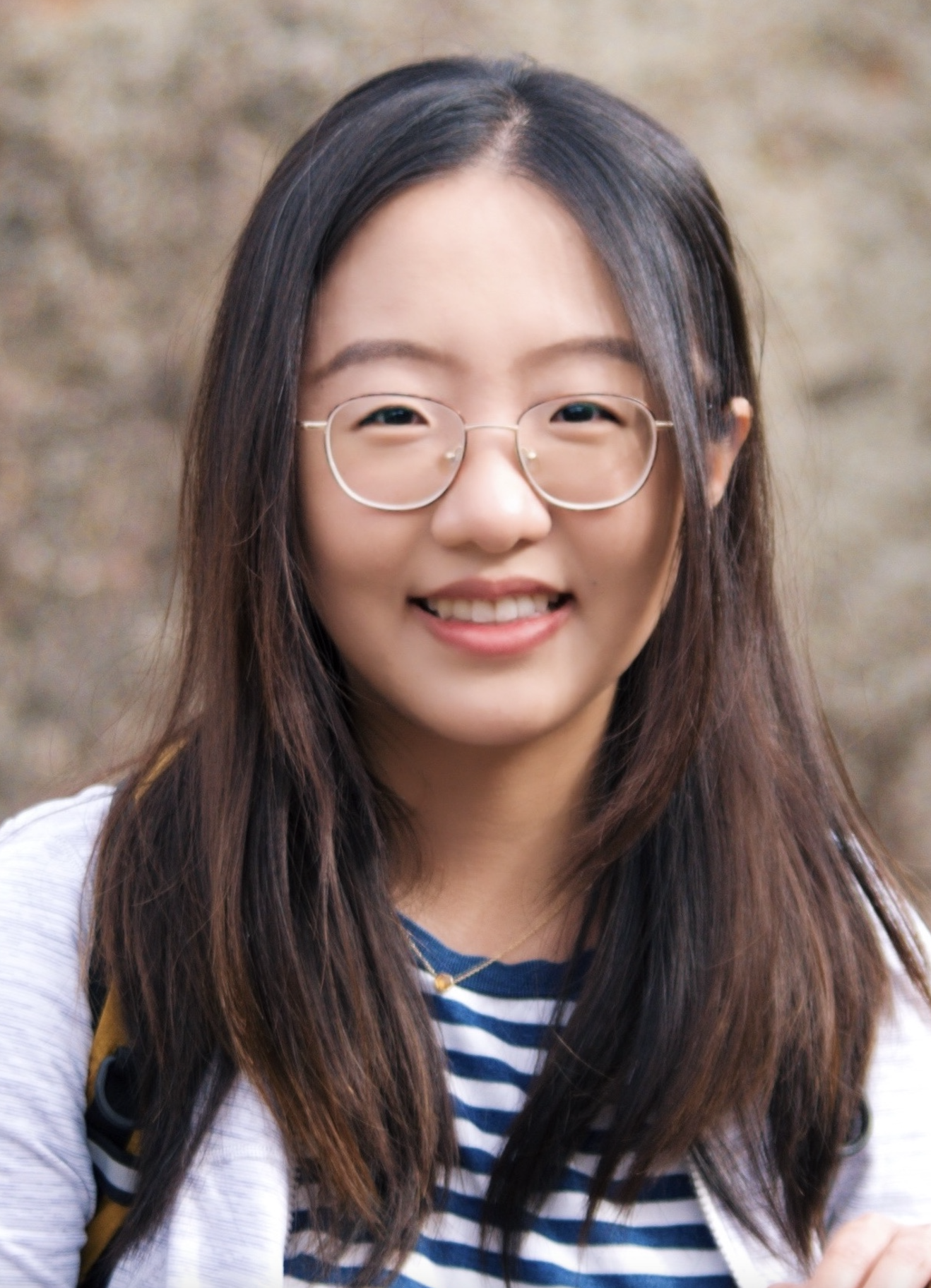
AI4Science Team
- Yuanqi Du (Cornell)
- Payal Chandak (MIT)
- Daisy Yi Ding (Stanford)
- Chenru Duan (MSFT Quantum)
- Ada Fang (Harvard)
- Tianfan Fu (UIUC)
- Wenhao Gao (MIT)
- Kexin Huang (Stanford)
- Shengchao Liu (MILA)
- Ziming Liu (MIT)
- Hanchen Wang (Stanford, Genentech)
- Max Tegmark (MIT)
- Adrian Weller (Cambridge)
- Max Welling (UvA)
- Marinka Zitnik (Harvard)
- Connor Coley (MIT)
- Le Song (BioMap)
- Linfeng Zhang (DP Technology)
- Anima Anandkumar (Caltech, NVIDIA)
- Yoshua Bengio (MILA, Université de Montréal)
- Carla P. Gomes (Cornell)
- Aviv Regev (MIT, Genentech)